Ethical AI: Navigating Bias and Fairness in Machine Learning
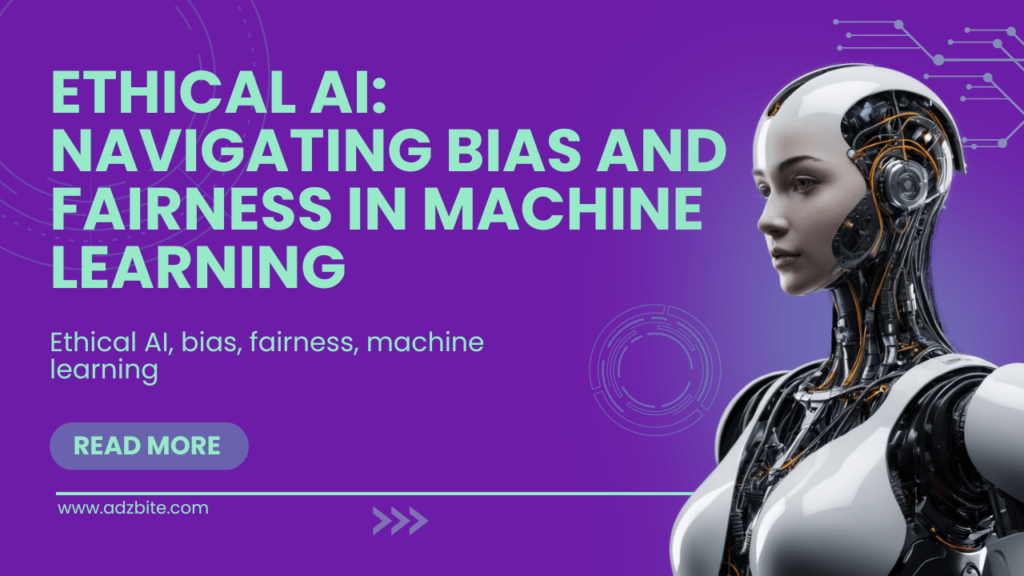
Explore the complexities of Ethical AI: Navigating Bias and Fairness in Machine Learning in this comprehensive guide. Uncover strategies, insights, and expert advice to ensure AI systems are developed and used ethically.
Introduction
In the realm of Artificial Intelligence (AI), the conversation around ethics, bias, and fairness is gaining momentum. As AI technologies become more integrated into our daily lives, ensuring they are developed and utilized ethically is paramount. This article dives deep into the landscape of Ethical AI, focusing on Navigating Bias and Fairness in Machine Learning.
Ethical AI: Navigating Bias and Fairness in Machine Learning
Artificial Intelligence (AI) has the power to revolutionize industries, streamline processes, and enhance decision-making. However, with this power comes the responsibility to ensure AI systems are developed and deployed ethically. In this article, we explore the nuances of Ethical AI, particularly focusing on Navigating Bias and Fairness in Machine Learning.
Understanding Ethical AI
Ethical AI encompasses principles and practices that guide the development and deployment of AI systems with fairness, accountability, and transparency in mind. It involves recognizing biases, addressing societal impacts, and upholding ethical standards throughout the AI lifecycle.
Defining Ethical AI
Ethical AI refers to the integration of ethical principles into the design, development, and use of AI technologies. It aims to mitigate potential harms and ensure AI systems align with societal values.
Importance of Ethical AI
In today’s interconnected world, AI decisions can have far-reaching consequences. Ethical AI is crucial for building trust among users, avoiding discriminatory outcomes, and promoting inclusivity.
Bias in Machine Learning
Machine Learning (ML), a subset of AI, relies on data to make decisions and predictions. However, biases present in training data can lead to skewed outcomes, reinforcing existing inequalities.
Types of Bias in ML
- Algorithmic Bias: Occurs when the ML algorithm’s assumptions or data lead to discriminatory results.
- Selection Bias: Arises when the training data does not adequately represent the entire population, leading to skewed predictions.
- Implicit Bias: Reflects the unconscious prejudices present in data collection and model development.
Impact of Bias in AI
Biased AI systems can perpetuate social injustices, amplify stereotypes, and exclude certain groups from opportunities. Recognizing and mitigating bias is essential for creating equitable AI solutions.
Fairness in AI Systems
Ensuring fairness in AI involves designing algorithms and systems that treat all individuals fairly and without discrimination. It requires proactive measures to prevent biased outcomes.
Ensuring Fairness in Algorithms
Fairness metrics, such as demographic parity and equal opportunity, can help assess and mitigate bias in AI models. Regular audits and transparency in AI decision-making processes are vital for accountability.
Addressing Unfair Outcomes
When unfair outcomes are detected, it is crucial to have mechanisms in place for corrective actions. This includes reevaluating training data, adjusting algorithms, and involving diverse perspectives in AI development.
Ethical AI Guidelines
Industry organizations and experts have developed guidelines and frameworks to promote ethical AI practices.
Industry Standards
Organizations like the IEEE and the AI Ethics Guidelines Global Network provide comprehensive frameworks for ethical AI development. These standards emphasize transparency, accountability, and inclusivity.
Best Practices for Ethical AI Implementation
- Diverse Teams: Building AI teams with diverse backgrounds and perspectives can uncover biases and foster innovation.
- Continuous Monitoring: Regularly evaluating AI systems for biases and fairness ensures ongoing ethical compliance.
- User Education: Educating users about AI capabilities and limitations promotes responsible use and informed decision-making.
Case Studies
Real-world examples highlight the successes and challenges of implementing Ethical AI.
Ethical AI Success Stories
- Healthcare: AI-driven diagnostics that prioritize patient privacy and equitable healthcare access.
- Finance: Fair lending models that reduce discriminatory practices and promote financial inclusion.
Lessons Learned from Ethical Failures
- Facial Recognition: Instances where biased facial recognition systems led to wrongful identifications.
- Recidivism Prediction: Biased algorithms in criminal justice systems resulting in unfair sentencing.
FAQs
Ethical AI refers to the integration of ethical principles into the development and use of artificial intelligence technologies.
Ethical AI is crucial for building trust among users, avoiding discriminatory outcomes, and promoting inclusivity in AI applications.
Bias in AI can lead to skewed outcomes, perpetuate social injustices, and exclude certain groups from opportunities.
Algorithmic bias, selection bias, and implicit bias are common types of biases found in Machine Learning models.
Ensuring fairness in AI involves designing algorithms that treat all individuals fairly and proactively mitigating bias through regular audits and transparency.
Best practices include forming diverse AI teams, continuous monitoring for biases, and educating users about AI limitations.
Conclusion
Ethical AI is not merely a concept; it is a call to action for developers, policymakers, and users alike. Navigating Bias and Fairness in Machine Learning requires a collective effort to build AI systems that reflect our values of equity and justice. By understanding the nuances of Ethical AI, we pave the way for a more inclusive and responsible AI-driven future.